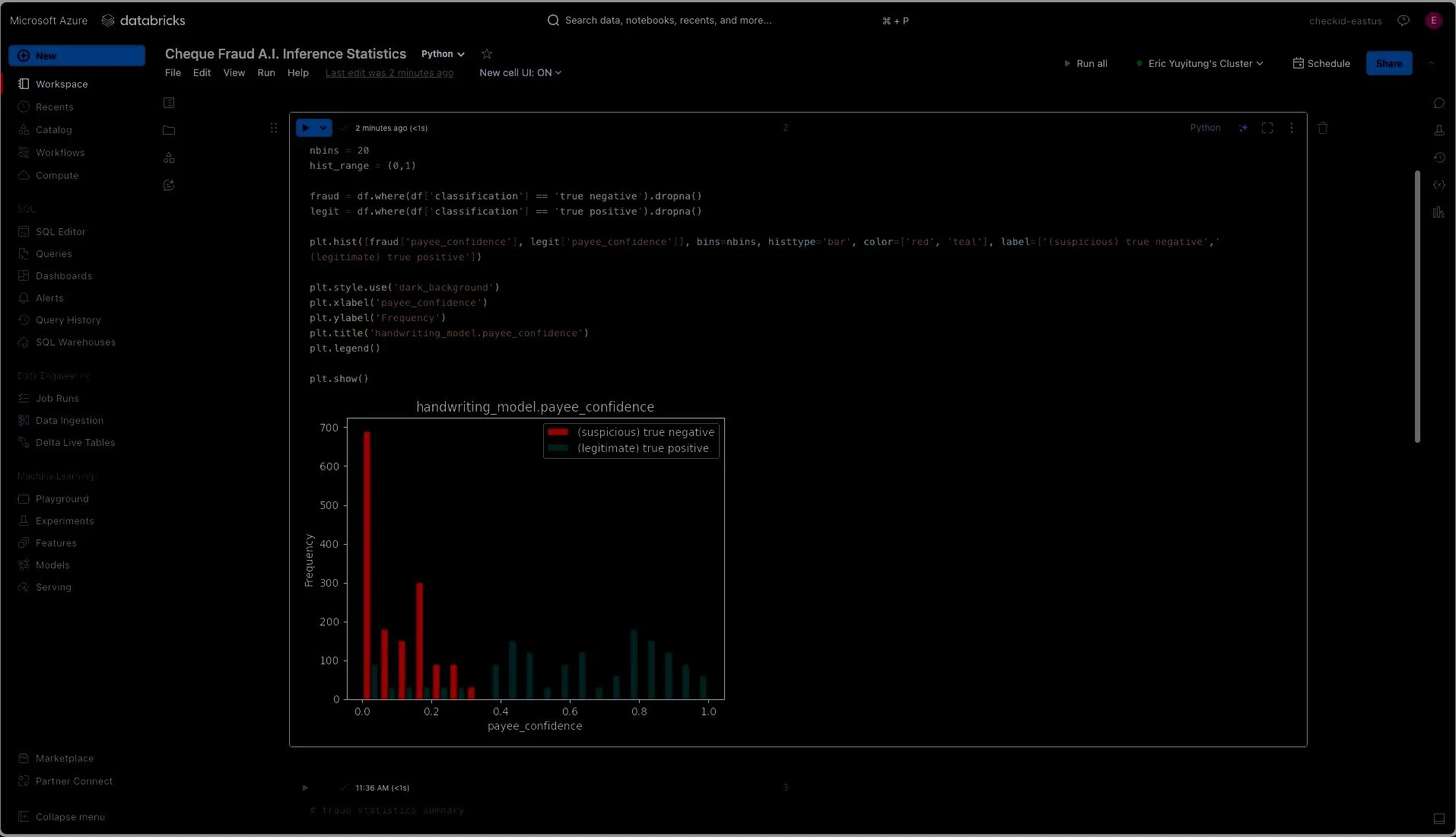
Data + A.I.
with Databricks
Analytics Optimization. Data Governance. AIOps.
INSIGHTS from A.I.
As a trusted engineering partner of Databricks, we have a proven track record in designing optimized Databricks solutions for Fortune 500 companies for their massive Data Lake. But what sets us apart from the others is our deep A.I. expertise in Databricks. Our A.I. research and engineering team has helped many organizations deploy and manage sophisticated A.I. models in inference, training, and drift monitoring to ensure peak performance and precision.
Optimized Streaming & Batched Processing
With Databricks and Azure Data Lake Storage (ADLS Gen2), we use the delta file format to store data across multiple zones with tailored retention policies, enhancing data management and performance. The landing zone retains batch files in their original formats. In contrast, the raw zone optimizes streaming data via partitioning and advanced settings to handle volume surges and improve read efficiency. Using techniques like VACUUM for file management and Change Data Feed (CDF) for temporal analytics enhances our data handling capabilities. Key strategies like delta format, table partitioning, and Databricks-specific configurations like storage caching ensure optimal performance.
data governance in lakehouse
Common challenges in data governance on data lake platforms, such as insufficient fine-grained access controls and a need for vendor-neutral solutions, are addressed by Databricks. The new Unity Catalog and legacy Table Access Controls (TAC) provide robust access management at the metastore level, including catalogues, schemas, views, and tables. These features enable column and row-level controls and custom data masking and serve as a unified platform for data cataloging, access management, auditing, lineage management, and data sharing across various solutions and cloud vendors.
Unity Catalog
Unity Catalog (UC), Databricks' latest feature, addresses former system constraints. It provides a detailed Data Catalog for a range of objects, facilitates registration of external data sources through the 'Lakehouse Federation'’ and offers native access controls. UC also has built-in lineage tracking, compatibility with hive API-supporting platforms, and delta-sharing capabilities for broad, secure data distribution. It has enhanced auditing features and ensures consistent access management across multiple Databricks workspaces in a single region.
ENTERPRISE A.I.
Databricks provides an extensive MLOps framework to enhance the machine learning lifecycle, encompassing development, deployment, and monitoring. It features automated model deployment using Python, notebooks, and SQL, executed through Azure Data Factory for consistency. Although it allows flexible development with its toolkit, it does not impose a standardized ML processing framework, offering flexibility. Model lineage is tracked with Unity Catalog and MLFlow, categorizing models by versions and tracking detailed experiment data. The model serving capabilities include real-time inference with autoscaling and configurable endpoints for A/B testing, with upcoming GPU support and integration with Azure ML available. Monitoring utilizes configurable SQL dashboards for comprehensive oversight of model performance and health, with support for custom metrics;
contact us
For top-tier Databricks engineering services, including infrastructure design, deployment, performance tuning, and A.I. deployment, look no further. Our team is ready to craft a solution that caters to your unique needs, ensuring optimal performance of your Databricks platform.